I am a senior lecturer at the Department of Electronic and Telecommunication Engineering at the University of Moratuwa, Sri Lanka. I work in the area of computer vision. Within this, surveillance, scene understanding, tracking, and activity recognition are of particular interest. We extensively use deep learning for our work. Our current work includes learning in robotics, making deep networks more effective by exploring new architectures, developing new routing algorithms, and improving convolution layers.
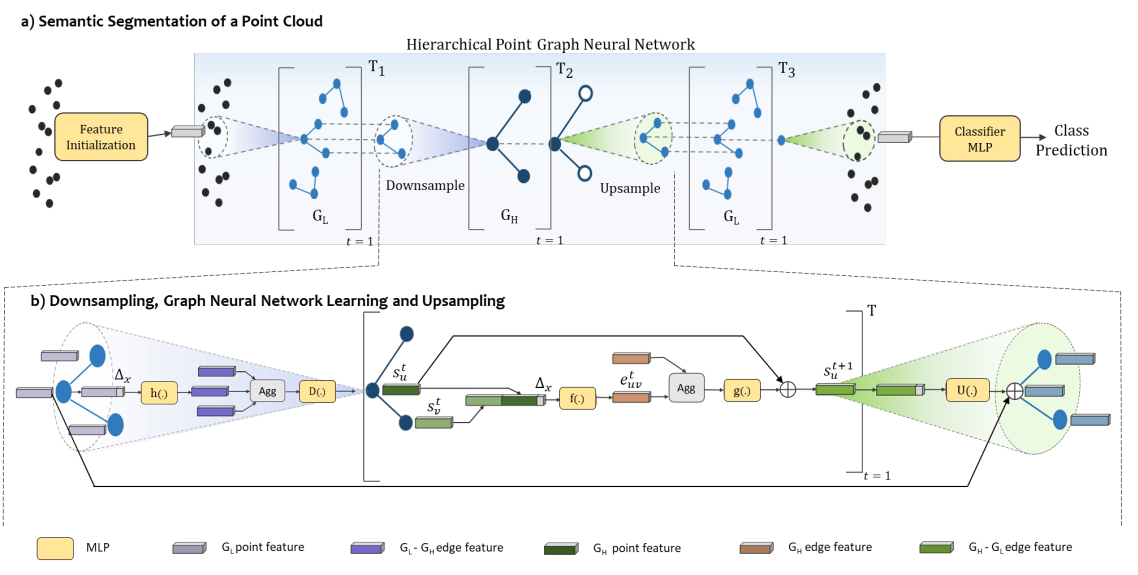
Point Cloud Processing
Point clouds, usually obtained using LiDARs, is an important perception manner commonplace in vision based autonomous navigation. One challenge of large-scale outdoor LiDAR point clouds is the high volume of points; generally millions of points per frame of observation. Object detection, and semantic segmentation important problems in the point-cloud domain.
Mohamed Afham, Isuru Dissanayake, Dinithi Dissanayake, Amaya Dharmasiri, Kanchana Thilakarathna, and Ranga Rodrigo, "CrossPoint: Self-Supervised Cross-Modal Contrastive Learning for 3D Point Cloud Understanding
," in Proceedings of IEEE/CVF Conference on Computer Vision and Pattern Recognition, New Orleans, LA, June 2022, pp. 1-10.
URL: https://openaccess.thecvf.com/content/CVPR2022/html/Afham_CrossPoint_Self-Supervised_Cross-Modal_Contrastive_Learning_for_3D_Point_Cloud_Understanding_CVPR_2022_paper.html
URL: https://openaccess.thecvf.com/content/CVPR2022/html/Afham_CrossPoint_Self-Supervised_Cross-Modal_Contrastive_Learning_for_3D_Point_Cloud_Understanding_CVPR_2022_paper.html
Arulmolivarman Thieshanthan, Amashi Niwarthana, Pamuditha Somarathne, Tharindu Wickremasinghe, and Ranga Rodrigo, "HPGNN: Using Hierarchical Graph Neural Networks for Outdoor Point Cloud Processing," in Proceedings of International Conference on Pattern Recognition, Montreal, QC, 2022, pp. 1--7.
URL: https://arxiv.org/abs/2206.02153
URL: https://arxiv.org/abs/2206.02153
Dishanika Denipitiyage, Vinoj Jayasundara, Chamira Edussooriya, and Ranga Rodrigo, "PointCaps: Raw point cloud processing using capsule networks with Euclidean distance routing," Journal of Visual Communication and Image Representation, vol. 88, no. , pp. 103612, 2022.
URL: https://www.sciencedirect.com/science/article/abs/pii/S1047320322001365
URL: https://www.sciencedirect.com/science/article/abs/pii/S1047320322001365
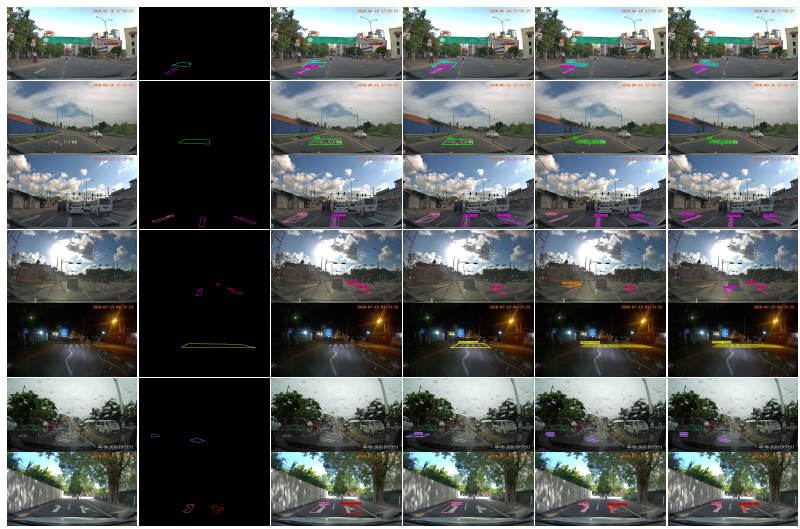
Vision for Self-Driving
Computer vision is an enabler for self-driving, whether the input is camera based-video or LIDAR-based point clouds. We are working along multiple avenues to contribute to this important area.
Road marking detection and lane detection directly assist self-driving. We have introduced a novel road marking benchmark dataset for road marking detection, addressing the limitations in the existing publicly available datasets such as lack of challenging scenarios, prominence given to lane markings, unavailability of an evaluation script, lack of annotation formats and lower resolutions. In SwiftLane, we presented a simple and light-weight, end-to-end deep learning based framework, coupled with the row-wise classification formulation for fast and efficient lane detection. Both these were with the support from Creative Software under the supervision of Dr. Peshala Jayasekara.
Inspired by recent improvements in point cloud processing for autonomous navigation, we focused on using hierarchical graph neural networks for processing and feature learning over large-scale outdoor LiDAR point clouds. In point based and GNN models for semantic segmentation with our work achieves a significant improvement for GNNs on the SemanticKITTI dataset. We have also made a more fundamental contribution in using cross-modal contrastive learning approach to learn transferable 3D point cloud representations.
Oshada Jayasinghe, Damith Anhettigama, Sahan Hemachandra , Shenali Kariyawasam , Ranga Rodrigo, and Peshala Jayasekara, "SwiftLane: Towards Fast and Efficient Lane Detection," in Proceedings of International Conference on Machine Learning and Applications, , 2021, pp. 1--6.
URL: https://arxiv.org/abs/2110.11779
URL: https://arxiv.org/abs/2110.11779
Oshada Jayasinghe, Sahan Hemachandra , Damith Anhettigama, Shenali Kariyawasam , Ranga Rodrigo, and Peshala Jayasekara, "CeyMo: See More on Roads - A Novel Benchmark Dataset for Road Marking
Detection," in Proceedings of IEEE/CVF Winter Conference on Applications of Computer Vision (WACV), Wikoloa, HI, January 2022, pp. 3104--3113.
URL: https://openaccess.thecvf.com/content/WACV2022/html/Jayasinghe_CeyMo_See_More_on_Roads_-_A_Novel_Benchmark_Dataset_WACV_2022_paper.html
URL: https://openaccess.thecvf.com/content/WACV2022/html/Jayasinghe_CeyMo_See_More_on_Roads_-_A_Novel_Benchmark_Dataset_WACV_2022_paper.html
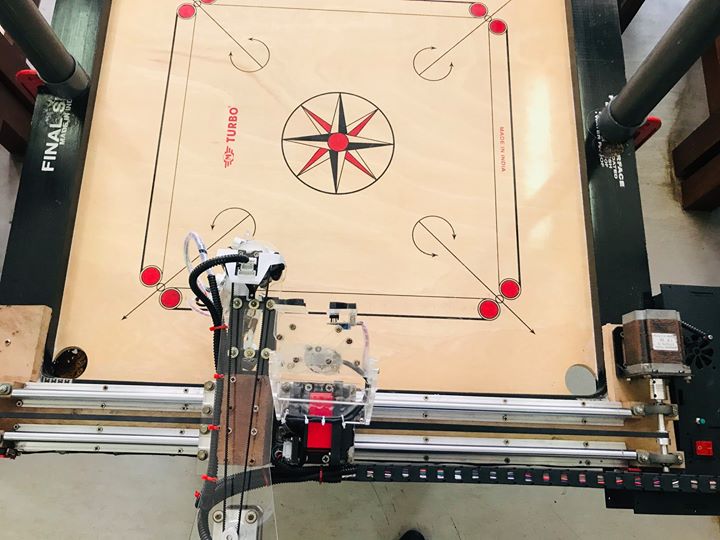
Board Games: Learning beyond Simulations
Reinforcement learning algorithms have been successfully trained for games like GO, Atari, and Chess in simulated environments. However, in cue sport-based games like Carrom, real world is unpredictable unlike in Chess and GO due to the stochastic nature of the gameplay as well as the effect of external factors such as friction combined with multiple collisions. Hence, solely training in a simulated platform for games like Billiard and Carrom, which need precise execution of a shot, would not be ideal in actual gameplay. This paper presents a real-time vision based efficient robotic system to play Carrom against a proficient human opponent. We demonstrate the challenges of adopting a reinforcement learning algorithm beyond simulations in implementing strategic gameplay for the robotic system. We currently achieve an overall shot accuracy of 70.6% by combining heuristic and reinforcement learning algorithms. Analysis of the overall results suggests the possibility of adopting a realworld training for board games which need precise mechanical actuation beyond simulations.
Naveen Karunanayake, Achintha Wijesinghe, Chameera Wijethunga, Chinthani Kumaradasa, Peshala Jayasekara, and Ranga Rodrigo, "Towards a Smart Opponent for Board Games:
Learning beyond Simulations," in Proceedings of IEEE International Conference on Systems, Man, and Cybernetics, Toronto, CA (virtual), 2020, pp. 1--8.
See research for more details on projects. See publications for a list of publications.